|
MAIN PAGE
> Back to contents
Modern Education
Reference:
Berezina T.N., Balan A., Zimina A.A.
Evaluation of the effectiveness of training and the use of neural networks to predict biological age.
// Modern Education.
2023. № 2.
P. 1-16.
DOI: 10.25136/2409-8736.2023.2.68981 EDN: ACBMNP URL: https://en.nbpublish.com/library_read_article.php?id=68981
Evaluation of the effectiveness of training and the use of neural networks to predict biological age.
Berezina Tatiana Nikolaevna
Doctor of Psychology
Professor of the Department of Extreme Psychology at Moscow State University of Psychology and Education.
123290, Russia, Moscow, nab. Shelepikhinskaya, 2a, room 508
|
tanberez@mail.ru
|
|
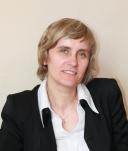 |
Other publications by this author |
|
Balan Aleksandr
MOU Princevskaya Secondary School
309980, Russia, Belgorod region, village. Printsevka, Sovetskaya str., 2
|
alexbalan@yandex.ru
|
|
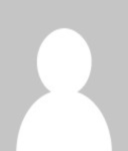 |
Other publications by this author |
|
|
Zimina Al'bina Aleksandrovna
ORCID: 0000-0003-3301-732X
Postgraduate student of the Department of Scientific Foundations of Extreme Psychology, Moscow State University of Psychology and Education
123290, Russia, Moscow region, Moscow, ul. Shelepikhinskaya Nab, 2a, office 207
|
leederx@mail.ru
|
|
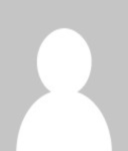 |
|
DOI: 10.25136/2409-8736.2023.2.68981
EDN: ACBMNP
Received:
09-11-2023
Published:
16-11-2023
Abstract:
Neural network training is widely used in various educational fields: staff training, mastering the curriculum at school and university, forming recommendations for private use by respondents, for teaching pensioners health-saving techniques. It is relevant to analyze the learning process of neural networks and evaluate their effectiveness on various models. For the study, a model was chosen for predicting the index of biological aging of a person based on the characteristics of his personality. To train neural networks, a data matrix of 1,632 people aged 35 to 70 years was compiled. Output variable: biological aging index; input variables: gender, age, family and professional status, place of residence, body type, type of functional asymmetry, style of relationships with people, as well as personal resources. Four neural networks were trained: for men and women, for working professionals and for pensioners. Results: 1) trained neural networks give different recommendations for men and women of pre-retirement and post-retirement age. 2) The effectiveness of predicting the biological aging index using neural networks turned out to be significantly high for all trained programs. 3) Neural networks can be used to model various social situations and identify what changes this will lead to for output variables. Situations were modeled: a) if all single adults get married, b) if all family adults break up, c) if everyone receives the recommendations of psychologists on the selection of personal resources and will use them. The neural network has issued a forecast: it is better for adult women not to change their family status/it is better for adult men to change their status. The use of personal resources selected by psychologists is effective for everyone.
Keywords:
training, automatic neural networks, neural network training, forecasting, personal resources, diagnostics, modeling, social situations, adult education, anti-aging
This article is automatically translated.
You can find original text of the article here.
Introduction Automatically neural networks are one of the options for the development of artificial intelligence and its application in various spheres of life. At the same time, since automatic neural networks are trainable systems, there is a problem of training neural networks and evaluating the effectiveness of this training, ethics and legality of using AI in various fields of activity [1]. In pedagogy, there are quite a large number of methods of teaching and evaluating learning outcomes. Some of these methods have found application in artificial intelligence programs being developed. Neural network training is based on the following algorithm. First, a large number of related input and output variables are introduced for training. Input variables are information that the user will subsequently enter, and output variables are possible responses generated by the neural network. Neural network training consists in the fact that the program itself finds patterns linking input and output variables (the neural network is learning). The trained neural network is checked for the adequacy of the identified patterns, usually another set of data is offered for this: a control (or test). On this set, it is checked to what extent the responses generated by the neural network coincide with the naturally received ones. It should be noted that currently all AI development programs are narrowly focused, the same applies to automatic neural networks. There are neural networks designed for drawing images from text [2], there are neural networks that answer questions on the topic [3], there are neural networks for predicting the effect based on the preliminary data entered [4]. This is how trained neural networks differ from trained people, who, as a rule, are able to perform a variety of tasks. As noted by domestic researchers, neural networks do not create new ideas and are not the creators of innovative breakthrough visual concepts [5], however, this does not prevent their use in pedagogy, which implements the task of translating life experience from generation to generation [6]. The development of Russian society is currently being carried out in the context of political and socio-economic transformations, the transformation of education and the development of digital technologies. The problem of neural network training is all the more urgent because it is a mutually similar process. Researchers train neural networks, and then trained neural networks find applications in various fields of science and practice, including pedagogy. Here are examples of the practical application of neural networks for training. Neural networks are used for staff training. The use of artificial intelligence and machine learning in personnel policy has become especially important. According to experts, in our country, many companies entrust the recruitment of personnel to trained neural networks, which allowed them to significantly optimize the recruitment process [7]. Neural networks are often used for teaching foreign languages. The ChatGPT neural network is most often used for these purposes, which, according to the authors, surpasses existing analogues in generating texts with specified parameters on a given topic. At the same time, this network is accessible to users, this has made it more popular compared to previous versions, there is currently an extensive discussion about using it in the educational process. Most authors believe that in order to use neural networks in teaching foreign languages, it is necessary to form at least superficial ideas about how modern automatic text processing systems work in natural language, in addition, the skill of interacting with these systems is necessary [3]. Currently, education in the field of art graphics is changing. There is a problem of how art education should develop in Russia due to the universal availability of generative neural networks. As you know, neural networks are able not only to change existing images, but even to create previously non-existent images. Teachers are concerned that an unprepared viewer cannot separate the works of a human author from the works of a neural network. In addition, the neural network has advantages, it can cope with visualization tasks faster than a person. E. L. Elkina believes that the existence of a huge amount of open data in the world of software reality poses a threat to the exclusivity of the human right to creative activity [8]. It indicates problems with the motivation of students in connection with technological development and . discusses the features and advantages of using automatic neural network technologies in teaching fine arts. Properly trained neural networks can solve the problem of forecasting [9] We consider it very important to train neural networks to solve problems of predicting human behavior or health [10]. It has its own specifics of training. The training of such neural networks is carried out on the principle of organizing neural networks of cells of living organisms. Such a system is not programmed at the development stage into the final consumable product, but is trained throughout its operation. Learning consists of finding the percentage relationship between neurons and input data, which ultimately leads to the identification of complex relationships between the data provided. Currently, it is proposed to use neural networks trained in this way for diagnostic purposes [11]. Doctors believe that the neural network copes much faster and more accurately with a large amount of information received at the input, and at the output can give a more accurate diagnosis, which will lead to the development of personalized medicine. A neural network with correctly written program code, together with the incoming up-to-date and verified information, will allow to find correlations of many statistical indicators in the field of healthcare with great accuracy [Data Science]. Trained neural networks can also be used to solve the problem of selecting effective stimuli for a particular person. Neural networks are used in psychology precisely to solve such problems (for example, this is the methodology called the "Hallucination Machine", which combines deep ultra-precise neural networks, panoramic videos, virtual reality to simulate biologically plausible "artificial" hallucinations to a specific person [12].
Neural networks can already be used to diagnose cardiovascular diseases based on the signals of the SCG. Domestic researcher N. S. Konnova believes that neural networks can help reduce mortality and improve the duration and quality of life of people with heart diseases, since they are more advanced diagnostic tools, and can help to detect and prevent diseases in a timely manner [13]. Such a wide spread of neural networks in various fields raises the question of teachers about understanding the mechanisms of learning, application and evaluation of the effectiveness of neural networks used to work with people, for example in the psychology of aging and anti-aging. The possibility of using psychological markers to assess the rate of biological aging has been repeatedly claimed by researchers [14]. However, the abundance of data obtained and the complexity in the aggregate analysis of a large number of variables makes this task difficult for ordinary statisticians and may require the use of neural networks. One of the interesting areas of reconciliation of neural networks is the selection of personal anti–aging resources for representatives of different age groups, taking into account the types of their individuality [15]. This is the model we chose for our study Organization of research The purpose of this study is to train neural networks to predict the biological aging index based on the socio-biographical and personal characteristics of respondents and to assess the prospects of trained neural networks to predict the dynamics of the biological aging index with changes in individual characteristics of a person. Research methods. 1. To train neural networks, the standard software package "Automatic neural Networks" from the set of standard statistical programs Statistica 12, the "Regression" method was used. The following parameters were set for creating and training a neural network. Network type: MPP. The minimum number of hidden neurons is 1. The maximum number of hidden neurons is 1. Networks for learning: 20. Networks to save: 5 (later, 1 network was selected, showing maximum compliance with the original data in the test study). Size of subsamples: random. The training network was built on 70% of the sample size, the control network on 15% and the test network also on 15% of the sample. 2. Output variable (analogous to the dependent in the neural network method): the index of biological aging. To calculate it, we used the Voitenko biological age estimation method. For further work, we used only one of the indicators of this method: the aging index (BV-DBV) - the difference between biological age and proper biological age. 3. Input categorical variables. To evaluate them , we used: Battery for assessing individual-personal and socio-biographical characteristics of a person: Socio-biographical data: gender (male, female), age ((35-56 (60)) – professionals, 56(61) – 70)) – pensioners), having a family (long-term marriage, divorce, single), children) no children – there are children), place of residence (rural area, city, capital), profession (realistic, research, artistic, social, entrepreneurial, conventional). Based on the questionnaire. - preferred style of interaction/communication (cooperation, rivalry, compromise)[1]. - body type (ectomorph (asthenic), endomorph (picnic), mesomorph (athletic), non-deterministic (harmonious). Based on anthropometric data. - type of functional asymmetry (right-handed, left-handed, ambidexterous). Based on self-assessment. - the type of emotionality according to V. M. Rusalov (communicative, intellectual, psychomotor)[2]. 4. Input continuous variables: personal resources. Questionnaire for the diagnosis of personal resources by T.N. Berezina[3] . 13 personal resources were allocated: 1) Sport, 2) Order, 3) Creativity, 4) Intelligence, 5) Subject Resource (Handmade), 6) Kindness (Altruistic resource), 7) Humor, 8) Spirituality, 9) Risk, 10) Communication, 11) Nature, 12) Achievements, 13) Optimism. As a result, 4 neural networks were trained. 1) Neural network for professional women (age range 35-55 years) with a training algorithm: BFGS 61, with the activation function of hidden neurons; hyperbolic and the activation function of output neurons: exponential. 2) Neural network for professional men (age range 35-60 years) with the training algorithm: BFGS 80, with the activation function of hidden neurons: hyperbolic and the activation function of output neurons: identical. 3) Neural network for retired women (age range 56-70 years) with a training algorithm: BFGS 34, with the function of activation of hidden neurons Logistic and the function of activation of output neurons — Logistic. 4) a neural network for retired men (age range 61-70 years) with a training algorithm: BFGS 42, with the activation function of hidden neurons - logistic and the activation function of output neurons — component. 5. Evaluation of the reliability of trained neural networks was carried out by calculating correlation coefficients. The correlation coefficient between the aging index predicted by the neural network and the one actually measured was calculated.
6. Prediction of the biological aging index for randomly selected groups of men and women. We have modeled several theoretical situations using neural networks trained by us. We simulated 3 situations for random 100 subjects from a group of professionals. 1 situation: if all single subjects have married. 2 situation: if all the family subjects are divorced. 3 situation: all respondents began to use the resources recommended to them (those resources that contributed to slowing down aging for representatives of their types), 1 point was added to these resources. 7. The program "Anti-Aging-XXI" for the selection of personal resources [16]. It was used for 10 randomly selected male professional respondents. Based on this program, personal resources were determined, recommended and not recommended by it. This data was used to model the situation 3 with the help of a neural network "if all men use psychological recommendations to slow down aging." Subjects. In total, 1,632 people aged 35 to 70 years took part in the study, including 840 women and 792 men. To obtain a generalized sample, residents of different regions of Russia were surveyed: Moscow and the Moscow region, Orenburg region, North Ossetia, Ufa and the Republic of Bashkortostan. The subjects were selected on a territorial basis. One territorial area in the surveyed locality (assigned to an educational or medical institution) was selected, a part of it was randomly selected and examined. The results of the study. Based on the proposed data matrix, we have trained neural networks. The neural network accounted for a number of the influence of the indicators we set (socio-biographical and personal resources) on the index of biological aging. The weight of each indicator was calculated. Indicators with a weight having a positive sign accelerate the biological aging of the body, indicators with a weight having a negative sign slow down aging. We have placed all the indicators in the "favorability" rows, at the top the indicators that slow down aging, and at the bottom – which accelerate. Table 1 shows the favorability series of indicators compiled by 1 and 2 neural networks. Table 1. Contribution (weight) of individual and personal indicators to biological aging of individuals for adult men and women (36-55 (60) years) Men | Women | Indicators | Weight values | Indicators | Weight values | Kindness | -8,57 | Creative type of profession | -10.71 | Risk | -4,56 | sport |
-2.98 | Creation | -2,05 | Kindness | -2.91 | Spirituality | -1,93 | Ambidextrous | -2.63 | Progress | -1,86 | achievements | -1.76 | Conventional type of professions | -1,71 | humor | -1.53 | Ambidextrous | -1,65 | communication | -1.13 | sport |
-1,55 | Asthenic | -0.80 | Intellectual type of professions | -1,42 | Accommodation in the village | -0.62 | married | -1,12 | Intellectual emotionality | -0.56 | communication | -0,75 | Accommodation in the city | -0.56 | Indeterminate body type | -0,36 | Having children | -0.50 | Accommodation in the city | -0,34 | Creation |
-0.47 | Without children | -0,32 | Cooperation | -0.40 | picnic | -0,14 | Not married | -0.39 | Intellectual emotionality | -0,12 | athlete | -0.38 | Not married | 0,03 | Without children | -0.38 | Right-sided asymmetry | 0,19 | Activity | -0.37 | Compromise |
0,34 | Compromise | -0.33 | Rivalry | 0,41 | Subject emotionality | -0.29 | Order (control) | 0,43 | nature | -0.26 | Subject emotionality | 0,43 | Married | -0.26 | Cooperation | 0,45 | Divorced | -0.23 | Living in rural areas | 0,61 | Rivalry |
-0.09 | Asthenic | 0,80 | Order (control) | -0.08 | Communicative emotionality | 0,91 | Communicative emotionality | -0.07 | Athlete | 0,91 | Right- sided asymmetry | 0.00 | Social type of professions | 0,98 | Indeterminate physique | 0.17 | Realistic type of professions | 1,22 | Picnic | 0.18 | Accommodation in the capital |
1,45 | Accommodation in the capital | 0.35 | Having children | 1,57 | intelligence | 0.62 | optimism | 2,16 | Intellectual type of professions | 0.93 | Professional Type: Enterprising | 2,16 | spirituality | 1.50 | lonely | 2,30 | risk | 1.64 | humor | 2,51 | left - sided asymmetry |
1.82 | Left- sided type of asymmetry | 2,71 | Conventional type of professions | 1.86 | nature | 3,12 | Realistic type of professions | 2.044 | Intelligence | 4,86 | Optimism | 2.32 | Activity | 7,07 | Social type of professions. | 2.52 | As can be seen from the table, the favorability series of men and women constructed by the neural network differ. Women most often manifest: Sports, Kindness, Subject resource, Humor, Sociability, Personality traits are most often manifested: Professional type: Artistic, Type of right-left asymmetry: ambidextrous, Body type: asthenic, Type of residence: rural, Type of emotionality: intellectual. In men, the most preferred personal qualities are: Kindness, Risk, Creativity, Spirituality, Subject resource, and the most preferred personal qualities are: Professional type: The usual type of right-left- bilateral asymmetry: ambidextrous Professional type: Researcher Type of family: married Body type: indefinite. To assess the reliability of the neural network at the final stage, the correlation is calculated between the theoretical indicators calculated using a trained neural network and the initial empirical values of the indicator. We evaluated the reliability of the neural network we trained. Correlation coefficients were calculated between the actual values of the biological aging index and the outputs obtained using training and test neural networks. Table 2. Correlation coefficients between the actual values of the biological aging index and the outputs obtained with the help of training and test neural networks from professionals.
| Aging Index - Learning Neural Network | Aging Index - A control neural network | Aging Index - Test neural network | Men | 0,68*** | 0,56*** | 0,69**** | Women | 0,70*** | 0,63*** | 0,63*** | P<0.001 As can be seen from the table, the neural networks trained by us give a reliable forecast of biological aging in terms of the use of personal resources by a person and his individual typological features Table 3 shows the favorability series compiled on the basis of data provided by neural networks 3 and 4. Table 3. Contribution (weight) of individual and personal indicators to the biological aging of individuals for men and women of retirement age ((55 (60) - 70 years)). Men | Women | Indicators | Weight values | Indicators | Weight values |
Nature | -4,44 | Creation | -7,70 | Intellectual type of professions | -3,03 | Spirituality | -5,86 | Humor | -2,58 | Single (without family) | -5,18 | Risk | -2,13 | Intellectual type of professions | -4,52 | Subject emotionality | -1,83 | Progress | -3,81 | Asthenic | -1,76 |
Enterprising type of professions | -3,65 | Lonely | -1,36 | Asthenic | -3,61 | Intelligence | -1,26 | Optimism | -2,21 | Progress | -1,01 | Nature | -2,14 | Spirituality | -0,84 | Risk | -1,91 | Right - handed | -0,82 | Living in the city |
-1,84 | Having children | -0,69 | Having children | -1,75 | Realistic type of professions | -0,69 | Subject activity (needlework) | -1,33 | Family | -0,66 | Communication | -1,15 | Compromise type of interaction | -0,54 | Left - handed | -0,68 | Altruism (kindness ) | -0,47 | Cooperating type of interaction | -0,56 | Living in the capital |
-0,42 | Athlete | -0,49 | Living in the city | -0,39 | Artistic type of professions | -0,23 | Creation | -0,32 | With objective emotionality | -0,19 | Picnic | -0,29 | Compromise type of interaction | -0,14 | Childless | -0,18 | With social emotionality | 0,09 | Cooperating type of interaction | -0,13 |
Right - handed | 0,14 | Rival type of interaction | -0,11 | Intellectual classes | 0,15 | Living in the village | -0,05 | With intellectual emotionality | 0,41 | Left - handed | -0,01 | Living in the village | 0,46 | Ambidextrous | 0,00 | Humor | 0,61 | Enterprising type of professions | 0,01 | Ambidextrous |
0,88 | Social type of professions | 0,14 | Rival type of interaction | 1,00 | With intellectual emotionality | 0,36 | Indeterminate body type | 1,32 | Indeterminate body type | 0,58 | Social type of professions | 1,41 | With social emotionality | 0,62197 | Living in the capital | 1,69 | Athlete | 0,64 | Childless | 2,05 |
Order (Control) | 0,85 | Realistic type of professions | 2,06 | Conventional type of professions | 1,13 | Family | 2,62 | Divorced | 1,20 | Divorced | 2,83 | Artistic type of professions | 1,62 | Altruism (Kindness) | 2,95 | Communication | 1,88 | Picnic | 3,11 | Subject activity (needlework) | 1,96 |
Order (control) | 4,90 | Sport | 4,46 | Conventional type of professions | 5,28 | Optimism | 7,90 | Sport | 6,25 | As can be seen from the table, according to the neural network, the biological aging of retired women slows down: creativity, spirituality, achievements, optimism, nature, risk, needlework (subject activity), communication. Personality types: intellectual type of professions, enterprising type of professions, asthenic, living in the city, having children, lefty, cooperating type of interaction, athlete, artistic type of professions, with objective emotionality, compromise type of interaction. Indicators with a positive weight (all others) increase the index of biological aging. Men slow down aging: nature, humor, risk, achievements, spirituality, intelligence, altruism (kindness), creativity. Types of personality: intellectual type of professions, with objective emotionality, having children, realistic type of professions, compromise type. Other indicators accelerate aging. We evaluated the reliability of the trained neural networks by calculating the correlation coefficient between the aging indices predicted by the neural network and the ones actually obtained. The results are shown in table 4. Table 4. Correlation coefficients between the target variable (biological aging index) and indicators calculated using different types of neural networks in women and men. 1.MLP 40-1-1 | Aging Index - Learning Neural Network | Aging Index - A control neural network | Aging Index - Test neural network | Women | 0,69** |
0,67** | 0,63** | Men | 0,87** | 0,82** | 0,84** | p<0,01 As can be seen from Table 2, all indicators calculated using a trained neural network reliably correlate with empirically obtained indicators. Analysis of theoretical models modeled by neural networks. We have modeled several theoretical situations using neural networks trained by us. We simulated 3 situations for random 100 subjects. 1 situation: if all single subjects have married. 2 situation: if all the family subjects are divorced. Situation 3: if all subjects were given recommendations to increase favorable resources and they followed the recommendations, only low indicators increased, i.e., subjects who initially had only 0-3 points of resources were added 1 point to each resource. For men, we also modeled the situation with a decrease in non-recommended resources and with an individual selection of resources (for 10 people). The neural network trained by us simulated situations of changing marital status for the 1st series of the study and gave the following forecast. If all women get married, their average biological aging index will increase by 1.9 years, i.e., for modern single Russian women of the age of middle maturity (35-55 years) marriage will be a resource-intensive type of activity, it will increase the load on the body and will not give advantages. However, the reverse situation will not be favorable. If all married women get divorced, their biological aging index will increase by 0.7 years on average, i.e., they will also age. This may be due to the fact that changing the status of a married woman to the status of a divorced woman also leads to a load on the body and a waste of biological resources. The most positive neural network for women considered the use of personal resources for bioenergetic potential. If women use more personal resources that are favorable for them (Sports, Kindness, Subject resources), then their biological aging will slow down by an average of 3.2 years compared to the current one, they will feel younger and the body's health indicators will also improve. For men, the situation is more complicated. According to the forecast of the neural network, divorce turns out to be the most favorable for married men (they are 1.2 years younger), and for single men – marriage (they are 0.1 years younger). Unlike women, for whom everything is the opposite: both single marriage and family divorce lead to an increase in the biological age. This can be interpreted in such a way that in a long-existing marriage (possibly originated before the period under study, most likely, it is a marriage with growing children)) the load falls more on men, so for them divorce turns out to be a factor of rejuvenation, and for women of aging. However, the emergence of new marriages at the age of middle maturity leads to an increase in the workload of women, so they begin to age faster, and men, respectively, slower. The neural network also showed that group selection of personal resources for men is not effective. When we increased the level of use of favorable resources in the male sample (kindness, risk, creativity), the indicator of aging increased. But when we reduced the level of use of unfavorable resources (nature, intelligence, achievements), the aging rate still increased. We believe that this is because it is difficult to find personal resources favorable for all men at once. Probably, each man needs to select resources individually, taking into account all his typological features. With the help of a neural network, we modeled such a selection for 10 randomly selected men who initially had an increased aging index. The selection was based on recommendations for slowing down aging, obtained with the help of the Anti-Aging- XXI program. We reduced the resources not recommended by this program and increased the recommended ones. As a result of such modeling, the aging index decreased by 4.8 years. This means that men can also find resources that will help slow down their aging. But you need to do it individually, taking into account its typological characteristics, which corresponds to general characteristics of men [17]. Research prospects: It should be noted once again that all the situations discussed in this section are not actually received data. These are theoretical situations modeled by means of neural networks trained by us. In this section, we wanted to emphasize the capabilities of neural networks to predict the impact of various social situations (divorces, marriages, childbirth, relocation, etc.) on the general aging of the population. As well as the prospects of using previously trained neural networks for group or individual selection of personal resources for a person. In the future, neural networks can be trained in order to select personal resources for an individual to compensate for the negative effects of certain social changes in his life. Limitations of the results obtained. Of course, the effectiveness of the predictions made by the neural network needs additional research, since so far this is data generated by trained neural networks. Conclusion.
A popular technique related to artificial intelligence technologies – automatic neural networks, is a rather complex process. Modern programs using the principle of neural networks are characterized by a narrow focus; neural networks are specialized in performing one specific function and training is possible only within the framework of the application of this function. As a rule, training takes place on a large initial data matrix, in which input and output variables are present. Neural network training is the identification of an optimal algorithm for the connection of input (user request) and output (neural network response) variables. We have studied the possibilities of using the neural network method to predict the biological aging index based on socio-biographical and personal characteristics of a person. To train neural networks, a data matrix of 1,632 people aged 35 to 70 years was compiled. The index of biological aging was set as the output variable, and the input variables were personality indicators: gender, age, family and professional status, place of residence, body type, functional asymmetry, style of relationship with people, as well as personal resources that a person uses. Four neural networks were trained for men and women of different age groups. It has been shown that personality indicators that contribute to slowing down aging differ both for men and women, and for representatives of different age groups (working professionals and pensioners). The effectiveness of predicting the biological aging index based on personality data turned out to be significantly high for all trained neural networks. Trained neural networks differ from other data processing methods in that they can be used to simulate various social and personal situations. Using neural networks trained for working professionals (age group 36- 55(60) years) we modeled 3 situations and studied the possible change in the aging index of respondents. The situations were as follows: 1) if all married people got divorced, 2) if all single people got married, 3) if all people started using the recommendations of psychologists. The results were paradoxical. Married women are better off staying married, single women are better off staying single – in this case, they have a lower aging index. It was not possible to identify unambiguous patterns for men. But it is useful for all respondents to find out the personal resources that are suitable for them (for example, with the help of the Anti–Aging XXI program) and use them; according to the forecast of neural networks, this will help slow down their biological aging. Contribution of the authors: T.B. - general idea, selection of methods, research management, writing an article; A.Z. – data collection, participation in the discussion of results; A.B. - participation in data collection, matrix compilation, neural network training, forecasting, participation in the discussion of results. [1] Escape from Captivity game. Electronic resource New game - Escape from captivity (access date: 12.11.2023 [2] V.M.Rusalov Diagnostic methodology Electronic resource V. M. Rusalov Emotionality Diagnostic Methodology - Take an online test | Online Test Pad (access date: 12.11.2023) [3] Questionnaire of personal resources. Electronic resource https://onlinetestpad.com/z75qeipjpj5ck (access date: 12.11.2023)
References
1. Anokhin, K. V., Novoselov, K. S., & Smirnov, S. K. (2022). Artificial intelligence for science and science for artificial intelligence. K. V. Anokhin, K. S. Novoselov, S. K. Smirnov [etc.] (Eds.). Questions of Philosophy, 3, 93-105. doi:10.21146/0042-8744-2022-3-93-105
2. Frolov, V. A., Feklisov, E. D., Trofimov, M. A., & Voloboy, A. G. (2020). Synthesis of images of interiors for training neural networks. Preprints of IAM im. M.V. Keldysh, 81, 1-20. doi:10.20948/prepr-2020-81
3. Kogan, M. S. (2023). On the possible use of the chatgpt neural network in teaching foreign languages. M. S. Kogan. Foreign languages at school, 3, 31-38.
4. Grinshkun, V. V. (2007). Application of adaptive tests with neural networks in measuring the effectiveness of computer science education. V. V. Grinshkun, E. I. Goryushkin. Bulletin of Moscow State Pedagogical University. Series: Computer science and informatization of education, 10, 11-14.
5. Bulygina, A. O. (2023). The role of generative neural networks in teaching arts to students of art and graphic faculties. Problems of modern pedagogical education, 78-3, 44-47.
6. Berezina, T.N. (2013). On the emotional safety of the educational environment. Psychology and psychotechnics, 9, 897-902. doi:10.7256/2070-8955.2013.9.9375
7. Makhmetova, A. Zh. E. (2023). Neural networks in the personnel training system: problems and marketing prospects for application. A. Zh. E. Makhmetova, I. M. Kublin, R. O. Sharapov. Practical marketing, 4(310), 42-46. doi:10.24412/2071-3762-2023-4310-42-46
8. Elkina, E. L. (2023). Neural networks. AI in teaching composition to design students. Bulletin of Pedagogical Sciences, 3, 101-106.
9. Dianov, R. S. (2009). Training a neural network to predict the results of intensifying gas inflows in conditions of insufficient information. Bulletin of the Astrakhan State Technical University. Series: Management, computer technology and information science, 1, 101-104.
10. Gritskov, I. O., Govorov, A. V., & Vasiliev, A. O. (2021). Data Science-deep learning of neural networks and their application in healthcare. I. O. Gritskov, A. V. Govorov, A. O. Vasiliev [etc.]. Megapolis Health, 2(2), 109-115. doi:10.47619/2713-2617.zm.2021.v2i2;109-115
11. Petrov, A. N. (2018). Training a neural network as a tool for system analysis of multidimensional psychodiagnostic data. A. N. Petrov, G. F. Ivanova, E. V. Slavutskaya (Eds.). Bulletin of the Chuvash University, 1, 162-168.
12. Rastell,i C., Greco, A., Kenett, Y.N., Finocchiaro, C., & Pisapia, N. (2022). De Simulated visual hallucinations in virtual reality enhance cognitive flexibility. Scientific Reports. Vol. 12, article ID 4027. doi:10.1038/s41598-022-08047-w
13. Konnova, N. S. (2020). Application of neural networks and machine learning algorithms for the purpose of diagnosing cardiovascular diseases based on SCG signals / N. S. Konnova, V. Yu. Khaperskaya. Biomedical radioelectronics, 23(1), 5-20. doi:10.18127/j15604136-202001-01
14. Obukhova, L.F., Obukhova, O.B., & Shapovalenko, I.V. (2003). The problem of aging from biological and psychological points of view [Electronic resource]. Psychological science and education, 8(3).
15. Berezina, T.N., Litvinova, A.V., & Zinatullina, A.M. (2022). The relationship between individual and personal anti-aging strategies and biological age [Electronic resource]. Modern foreign psychology, 11(4), 73–89. Retrieved from https://doi.org/10.17759/jmfp.2022110407
16. Berezina, T.N. (2022). Certificate of state registration of a computer program No. 2022668987 Russian Federation. "Antiaging XXI": No. 2022668380: application. 10/07/2022: publ. 10.14.2022. T. N. Berezina, S. A. Rybtsov.
17. Finogenova, T. A. (2022). Emotional safety of the educational environment of a boarding school as a condition for reducing the destructive protective-coping behavior of its students. Human capital, 7(163), 174-184. doi:10.25629/HC.2022.07.19
Peer Review
Peer reviewers' evaluations remain confidential and are not disclosed to the public. Only external reviews, authorized for publication by the article's author(s), are made public. Typically, these final reviews are conducted after the manuscript's revision. Adhering to our double-blind review policy, the reviewer's identity is kept confidential.
The list of publisher reviewers can be found here.
The paper "Evaluation of the effectiveness of learning and the use of neural networks for predicting biological age" is submitted for review. The subject of the study. The work is aimed at training neural networks to predict the biological aging index based on the socio-biographical and personal characteristics of respondents and evaluating the prospects of trained neural networks to predict the dynamics of the biological aging index with changes in individual human characteristics. The work carried out made it possible to demonstrate its effectiveness. The goal was achieved. The research methodology is determined by relevance. The author conducted a theoretical and empirical study. It uses the neural network training method and confirms the effectiveness of the assumptions made. The relevance of the research is determined by the fact that it is currently important to find modern learning tools, including the training of automatic neural networks. The scientific novelty of the research. The conducted research made it possible to demonstrate the possibilities of using the neural network method to predict the biological aging index based on socio-biographical and personal characteristics of a person, as well as to obtain positive results. Style, structure, content. The style of presentation corresponds to publications of this level. The language of the work is scientific. The structure of the work is clearly traced, the author highlights the main semantic parts. In the introduction, the author noted the relevance of the problem raised. It was highlighted that automatic neural networks include trainable systems. In this regard, there is a problem of training neural networks and evaluating the effectiveness of this training, the ethics and legality of using artificial intelligence in various fields of activity. On the other hand, modern pedagogical science has a large number of teaching methods and evaluation of learning outcomes, some of which have found application in the developed artificial intelligence programs. The next section is devoted to the consideration of the algorithm for using neural networks in the learning process, and the description of the developed artificial intelligence programs. So, at present, neural networks are actively used for teaching foreign languages, personnel, artistic graphics, as well as for solving forecasting problems. The potential of using neural networks poses the problem of understanding learning mechanisms, applying and evaluating their effectiveness. One of the most interesting areas of reconciliation of neural networks is the selection of personal anti-aging resources for representatives of different age groups, taking into account their personality types, which is the model of the conducted research. Further, the author described the features of the organization of the study. The paper highlights the purpose, describes in detail the criteria ("variables") and research methods, as well as the respondents. As a result, four neural networks were trained: for professional women and men, as well as for women and men of retirement age. Special attention is paid to assessing the reliability of trained neural networks and predicting the biological aging index for randomly selected groups of men and women. The author actively used methods of mathematical statistics and correlation coefficients. The description of the Anti-Aging-XXI program for the selection of personal resources is important. The next section was devoted to the description and analysis of the results obtained. The author demonstrated the results of neural network training. The results are presented in the form of tables with a detailed description of the data obtained. The next section is devoted to the description of the analysis of theoretical models that have been modeled using neural networks. In total, 3 situations were created for random 100 subjects: - the first situation: all single subjects got married; - the second situation: all family subjects divorced. - the third situation: all the subjects were given recommendations to increase favorable resources and they followed the recommendations. The paper presents the results obtained, highlights the prospects of the research and the limitation of the results obtained. The article ends with a summary. Special attention in the results is devoted to the discussion of the following issues: - the possibility of using the neural network method to predict the biological aging index based on socio-biographical and personal characteristics of a person; - the difference between trained neural networks and other data processing methods; - the authors' contribution to solving the problem is highlighted. The conclusion, in general, contains reasonable and generalizing conclusions. Bibliography. The bibliography of the article includes 17 domestic and foreign sources, a significant part of which has been published over the past three years. The list contains mainly articles and abstracts. In addition, there are monographs, as well as a certificate of state registration of a computer program. The sources are designed, in general, correctly and uniformly. However, some positions need to be finalized, it is necessary to achieve uniformity in the design of articles (for example, issues 17 and 14). Appeal to opponents. Recommendations: - to carry out a more in-depth theoretical analysis of sources on the affected problem; - to correct the text for descriptions (For example, "Training neural networks to be built according to the following algorithm", etc.), missing text elements (For example, "... Training neural networks to be built according to the following algorithm ..."), etc. Conclusions. The problems of the article are of undoubted relevance, theoretical and practical value; it will be of interest to specialists who deal with the problems of learning using neural networks. The article can be recommended for publication taking into account the highlighted recommendations.
Link to this article
You can simply select and copy link from below text field.
|
|